Explore Our Latest Insights on Artificial Intelligence (AI). Learn More.
AI Security Risks and Recommendations: Demystifying the AI Box of Magic
by Alex Cowperthwaite, Pratik Amin
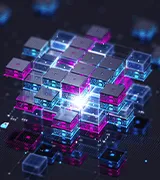
Thu, Jul 11, 2019
Explore Our Latest Insights on Artificial Intelligence (AI). Learn More.
This article outlines at a high level how artificial intelligence (AI) can help organizations proactively detect data breaches in their earliest stages in order to prevent them from escalating into major events. Machine learning is used to curate and extract meaningful information from the immense amount of data on the Deep Dark Web (DDW) by reducing and optimizing the search space. In this way, analysts can more easily identify risk markers for data losses. Sophisticated data visualization tools take this information, i.e., the exposure of PII or loss of other sensitive data, to create a timeline of events on the DDW leading up to a data security breach that can subsequently be used as the basis for an early warning system.
Regulators, consumers and investors/stakeholders are increasingly not willing to accept the prevailing “not if, but when” defeatist attitude regarding data breaches. For example, the commission set up to oversee implementation for the European Union’s General Data Protection Regulation (GDPR) unequivocally states, “As an organization it is vital to implement appropriate technical and organizational measures to avoid possible data breaches.”
So, it is not a rhetorical question to ask: What if organizations could predict, with a great degree of confidence, where and when their data might be compromised?
One of the key areas to find answers is in the deep dark web (DDW), with its known havens for cybercriminals and other bad actors. However, the DDW is a huge environment; not only does it have a decades-long history of data, but it also continues to grow at a staggering pace across a multitude of protocols, forums and sources.
Extracting relevant information from the DDW’s millions of files and petabytes1 of information is a herculean and complex challenge, but just one part of the threat intelligence equation. Today, researchers are exploring exciting new frontiers in artificial intelligence and machine learning to determine the data that is meaningful and to create useful models to advance cybersecurity efforts.
Having identified and indexed DDW data for more than 14 years, Kroll has created a comprehensive data resource that is continually refreshed and curated. In analyzing this wealth of data, researchers hypothesized that by understanding the patterns and contexts leading up to data breaches, threat intelligence experts could better predict where and when future breaches might occur with the goal of preventing these from escalating into major events.
This article describes a methodology that addresses the challenge for organizations in three phases:
Reduce and Optimize the Search Space With AI
The process begins by creating a list of key terms, IP addresses, domain names, etc., that are unique to the organization (Kroll calls this the Dynamic Signature Profile or DSP). Given the near-overwhelming vastness of the DDW, optimizing the search space is required to separate vital signals from the noise.
AI is used to remove files that may contain DSP terms but which do not represent a risk. These can include items such as large pdf books, media interviews, marketing collateral, speaking engagements, etc. This is accomplished by training several supervised machine learning models to distinguish between pertinent and irrelevant files. Current trained models developed by the firm achieve 99.97% accuracy on this task by combining an initial dataset of several thousands of files with insights from highly experienced threat intelligence analysts.
Finding Organization-Specific “Haystack Needles” in the DDW
After reducing the search space, the next step is to use cognitive clustering to find patterns across the reduced but still very massive data store.
Clustering approaches supplemented by interactive human judgment are fundamental to the firm’s process. Over time, files based on salient terms and metadata have been organized into coherent groups that reflect various aspects of exposure to potential data losses.
In this way, several productive patterns have been uncovered that can be routinely monitored by analysts using predictive analytics tools. This combination of man-machine analysis has helped find several valuable exposure indicators across different industries. For example, in one case, large numbers of travel itineraries for an airline were found in the DDW, which indicated a specific vulnerability for this organization.
Learning from the above analysis, similar techniques are applied to the relatively smaller set of files that are pertinent to a specific organization, enabling the discovery of risk indicators specific to them in the DDW datastore. As clusters emerge for a given organization, the exposure assessment model is applied to help determine the organization’s level of risk on the DDW.
The methodology used for this phase includes developing organization-specific exposure indicators that take into account context idiosyncrasies. These indicators are built on the basis of signals mined from the text, which analysts use to create a “salient term matrix.” Analysts also examine the format and review occurrence characteristics, such as the timing, protocols and locations of organization-pertinent files.
An active learning loop with human analysts continues to refine and expand these signals. This loop also helps optimize the number of clusters for human review, which enhances the analysts’ ability to find evidence pertinent to potential data loss events for an organization.
Proactively Detecting Data Loss Based on Files Related to an Organization Being Seen on the DDW
Once organization-specific exposure indicators and clusters have been determined, the next phase involves a timeline analysis to study historical pre-breach patterns with the goal of eventually predicting potential breaches utilizing AI. The basic premise of the model is outlined below.
Researchers have found that clustering analysis shows a significant increase in the number of files on the DDW for an organization after a data loss event. They also observed that bad actors will accumulate files and once a threshold of sufficient data is met, the information is exploited for potentially nefarious activities. For example, the chart below shows how clusters emerged for a given organization over a 10-year time period (source of data: Kroll’s DDW datastore). The third data loss event in the past five years ultimately gave bad actors enough exposure to act on the data.
Models trained in this way can detect the surge in activities for organization, which combined with active monitoring by human analysts, helps threat intelligence specialists to detect potential breaches. The goal is by leveraging automated systems, human team members can alert organizations as these clusters emerge. In Kroll’s case, it enables us to work with clients to proactively detect data loss before it becomes a larger incident and to help them understand where the data exposure is coming from within their organization.
The integration of Kroll’s investigative expertise with the next generation AI and machine learning into CyberDetectER DarkWeb can help organizations better understand and address their data’s exposure on the DDW. As researchers continue to refine and grow models and methodologies, they will be able to help organizations proactively detect data loss and prevent those data losses from escalating into major events that can harm their operations, finances and reputations for years to come.
A version of this article was previously published in Corporate Compliance Insights and GARP.
Sources
1 One petabyte = 250 bytes; 1024 terabytes, or a million gigabytes
Incident response, digital forensics, breach notification, security strategy, managed security services, discovery solutions, security transformation.
Kroll is the largest global IR provider with experienced responders who can handle the entire security incident lifecycle.
Services include drafting communications, full-service mailing, alternate notifications.
by Alex Cowperthwaite, Pratik Amin
by Mark Turner, Richard Taylor, Richard Kerr
by Ana D. Petrovic
by Ana D. Petrovic, Jack Thomas, Justin Hearon